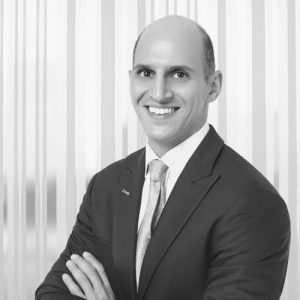
Advanced Analytics Insights
by Zaid Kamhawi, CEO, Qarar
In our second article exploring the impact of Artificial Intelligence (AI) in the banking and finance sector, Zaid Kamhawi discusses how AI can enable lenders to score potential borrowers on their creditworthiness.
This is a particularly hot topic — the use of AI in the context of creditworthiness — and there are several reasons for this. We already know that lending is the most important decision that a bank or a financial institution has to make because it is fundamentally core to its business. So, it is no surprise that lenders have invested heavily over the years in trying to use data analytics to be able to apply it to credit decisioning — ultimately to try to predict the creditworthiness of consumers.
These models that have been built to help predict creditworthiness, are typically built by analysing large amounts of data about past borrowers to try to identify relationships between variables and characteristics that can then be used to predict future behavior of similar borrowers. So, in a nutshell, that is how these predictive models have typically been built. Fast forward to the present day and there is more and more data becoming available about consumers. Therefore, it becomes increasingly difficult to utilise the traditional ways of model development to try to identify all the possible correlations between the myriad pieces of data about customers.
This is where AI has recently played a significant role — particularly in the context of machine learning — which is a sophisticated analytical technique and a big enabler of AI. Machine learning has the potential to develop superior predictive power and it is able to do that by its ability for advanced pattern recognition. Consequently, machine learning can identify relationships among thousands and thousands of variables that are not typically intuitive or easily revealed using traditional modeling techniques, especially when it comes to analysing what is known as alternative data.
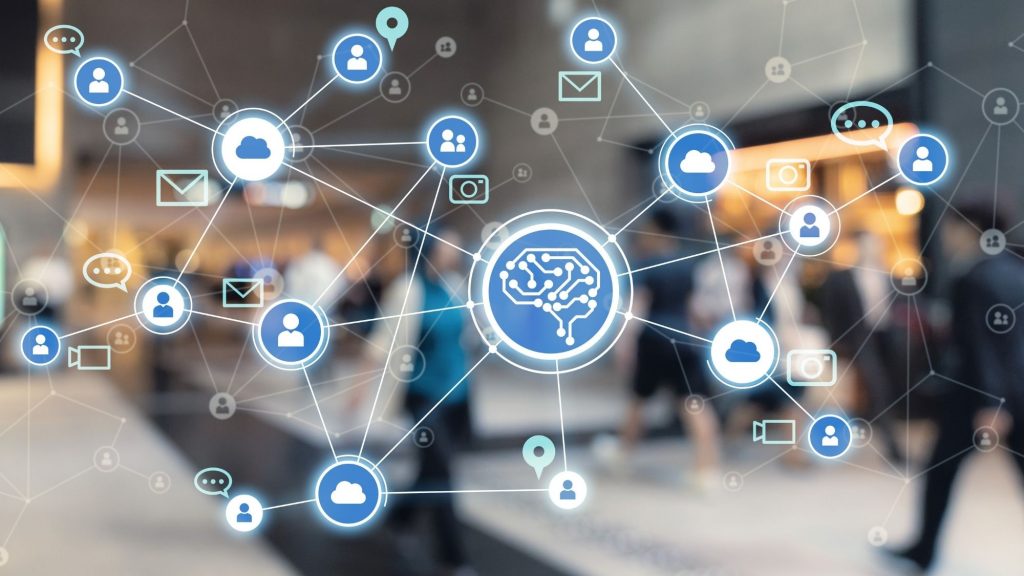
When we start looking at people’s social media data, telco information or device data, and try to use that type of information to link it to an individual’s credit risk, this is where AI and machine learning has come to play a major role. What AI really means to creditworthiness — or to the assessment of creditworthiness — is an explosion in the amount of data and information that can be used to more accurately determine the credit risk associated with an individual. This is vitally important. Even a one percent increase in that predictive power can mean millions of profitable decisions for banks. More importantly, it means access to credit can be expanded to more people, achieving financial inclusion. Obviously, it also helps in enhancing the accuracy of prediction of existing bank customers.
Therefore, it comes as no surprise to learn that 70% of all financial services firms were using machine learning to predict credit scores and predict fraud, according to a recent survey by Deloitte Insights. A similar survey revealed that 77% of banking institutions in the UAE stated that they plan to use AI to automate some form of tasks — not necessarily only credit assessment and creditworthiness.
In the Middle East, we have not seen as much take-up of AI or machine learning in the credit risk space as in other regions. There is probably a good reason for this; we have to remember that credit risk models were first established in order to address the bias from subjective decision making, and so a great deal of care needs to be taken not to replace one bias-prone approach with another. Models that are driven by machine learning, on one hand, are very highly predictive, but they are so sophisticated that they come at a price of opaqueness — they are seen as black boxes that nobody can explain.
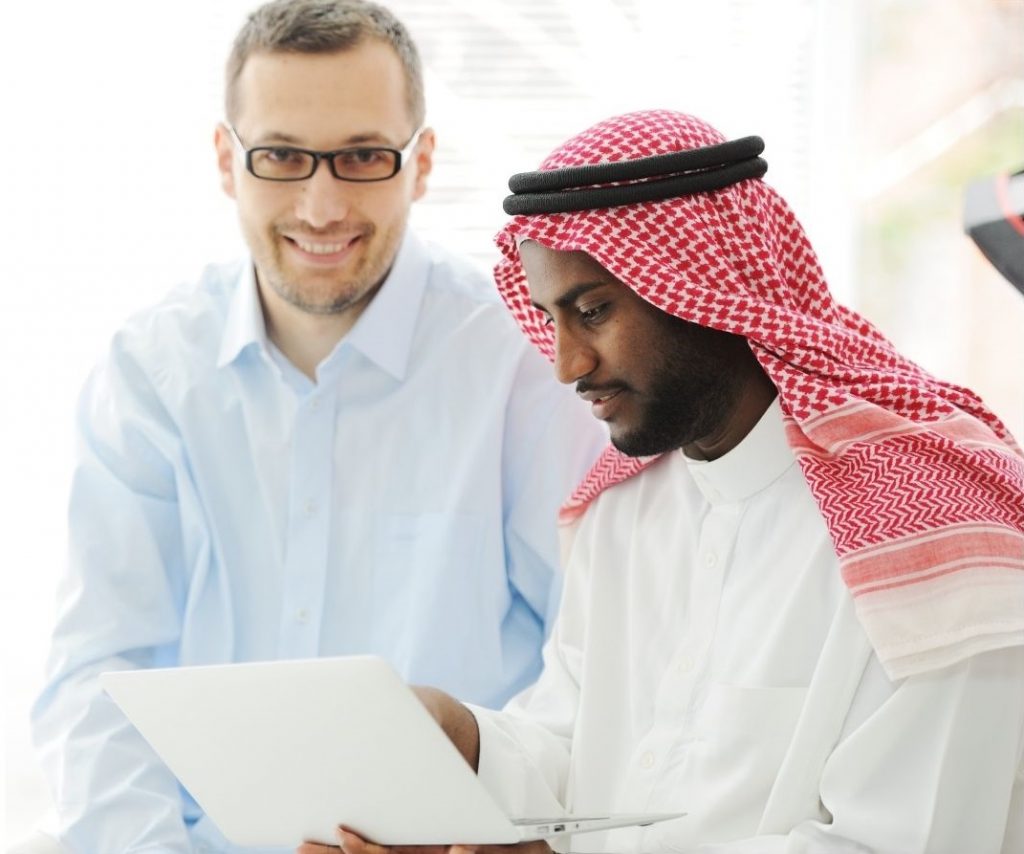
It is for this reason why the biggest challenge for today is how AI can be applied into credit models, but still make sure that these models are designed with fairness at their core. We have to remember that the overall purpose is not just to become more accurate, but also to continue to be more inclusive.
There have been great strides made in AI development. The advancements in explainable AI have now enabled these models to be more transparent that we can now expect to see a higher adoption of AI in assessing creditworthiness in the coming years.